Fully automated data curation
We provide a fully automated data curation service which can be used to enhance the quality of raw clinical, genetic and lifestyle data. The service supports functionalities for metadata extraction, outlier detection, data imputation, and de-duplication.
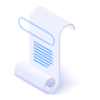
Flexible performance and scale
Both conventional methods, such as, the z-score and the interquartile range, as well as, advanced methods, such as, the isolation forests and the Gaussian elliptic curves are used to detect anomalies within the raw data.
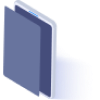
Advanced data imputers
Advanced data imputers are used to replace the missing values based on virtual patient profiles which are built for each real patient. Similarity detection methods and lexical matchers are used to identify variables with identical distributions and common meaning. Useful metadata are provided to the user along with detailed reports where the inconsistent fields are highlighted with color coding
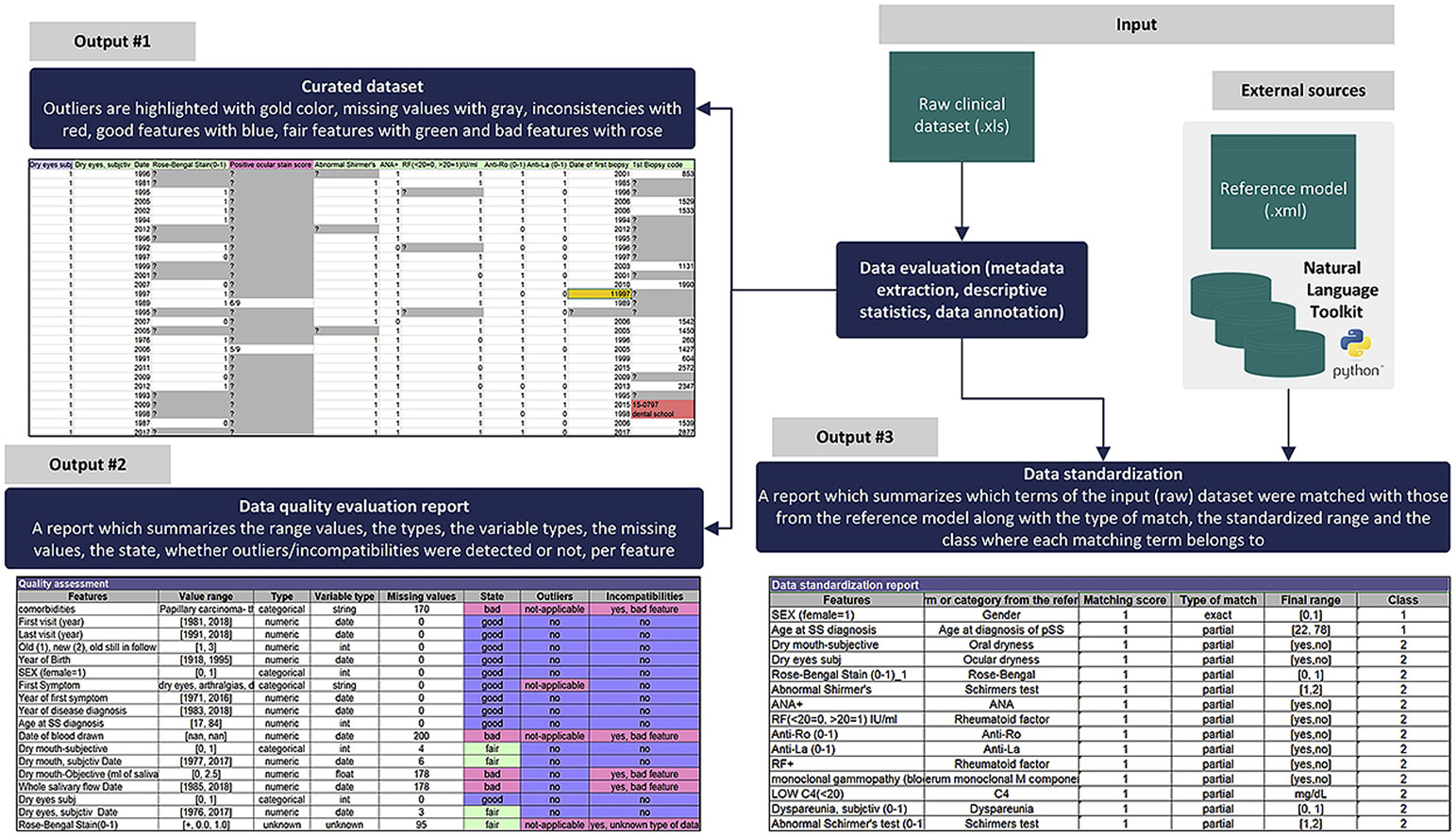
Pezoulas VC, Kourou KD, Kalatzis F, Exarchos TP, Venetsanopoulou A, Zampeli E, Gandolfo S, Skopouli F, De Vita S, Tzioufas AG, Fotiadis DI. Medical data quality assessment: On the development of an automated framework for medical data curation. Comput Biol Med. 2019 Apr;107:270-283. doi: 10.1016/j.compbiomed.2019.03.001. Epub 2019 Mar 7. PMID: 30878889.
Hybrid data harmonization
We have a long standing experience in handling data and cohorts from different clinical centers and in performing data harmonization.

Heterogenity of data
We offer a hybrid data harmonization service which uses advanced lexical and semantic analyzers to detect terminologies with both conceptual and lexical basis aiming to overcome structural heterogeneities across retrospectice medical data from diverse medical centers. To this end, we use WebProtege to transform conventional data models into ontologies (hierarchical data models) towards the definition of reference ontologies for different diseases with respect to the HL7 FHIR and FAIR principles.

Reference ontologies
The reference ontologies are enriched with word embeddings and additional terminologies from Python's NLTK and external vocabularies (e.g. OHDSI Athena) to define a large corpus. The corpus is finally deployed to identify terminologies with common conceptual and/or lexical basis in the raw databases per domain of interest and the values are standardized according to the clinical practice.
Our key differentiators

easy to use
The data harmonization workflow is depicted in Fig. 1 and consists of 4 stages, including the: (i) metadata extraction and relational modeling, (ii) construction of reference semantic data models (ontologies) for the CVD and mental disorders, (iii) development of medical dictionaries by interlinking the word embeddings from the ontologies with external knowledge repositories, such as, the OHDSI (Observational Health Data Sciences and Informatics) [15], and (iv) lexical and semantic analysis. The latter are built on top of the dictionaries to identify terminologies with lexical and conceptual basis. The output is a data harmonization report which includes the matching scores for each identified terminology along with useful metadata.